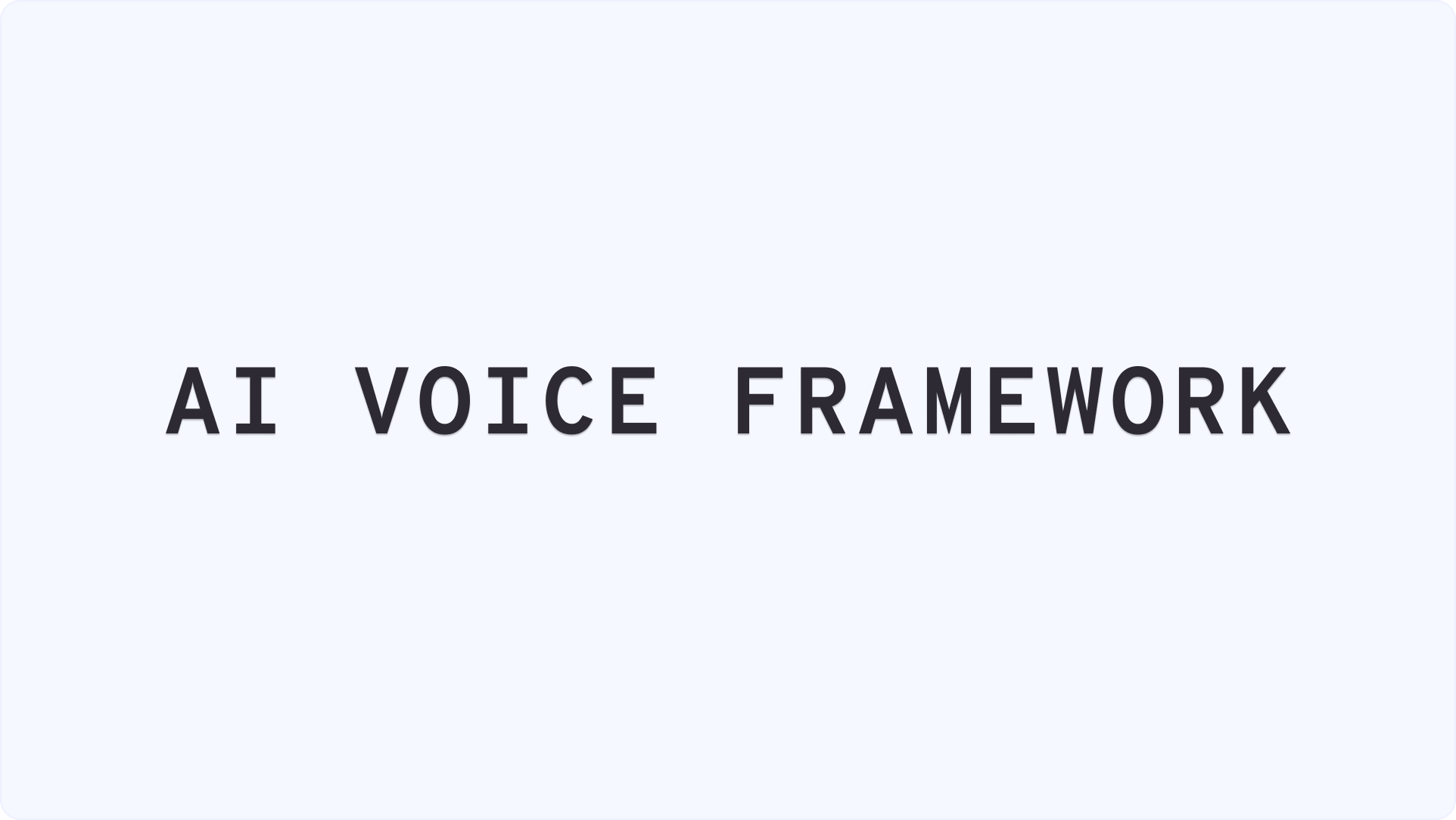
TIMELINE
Summer → Autumn 2024
USERS
Voice of Customer (VoC) analysts
Myself, author and designer
+ guidance from UXD, UXR, and product
TEAM
Qualtrics Assist:
An AI assistant that helps analysts get deeper insights from their data.
With Qualtrics Assist already built for the employee experience, we needed to make this work for our customers. The previous designer put together a voice settings flow, but the tone options didn’t match our standards.
AN EXPLORATION… OF AN EXPLORATION
Do these tones even make sense for QA in CX?
Why would a user choose a witty chat? We’re not even witty in our product
Personable and direct falls in line more with voice
Confident assumes that there is an unconfident tone
Again, this falls in line more with voice — we want users to trust in what we’re saying
Empathetic is standard
So I wondered,
Contrast with our voice and tone:
I first familiarized myself with UXR’s tonality study, asking how AI-output tone impacts user trust, desirability, and adoption.
GOALS
High level questions
Do different tones facilitate trust building? (Trait: Trust)
Does the tone of the feature or product impact the user’s willingness to adopt it? (Trait: Desirability)
Which tone builds a stronger emotional connection and prompts action?
→ Tone is fundamental to personalizing AI
Mirroring speech patterns between human and AI systems engenders more trust in AI (Kaplan et al., 2021)
A human-like persona can actually increase liking and trust (de Visser et al., 2017)
Trust can also be affected if the AI has no character, simply a function of its inputs (Dorton & Harper, 2022)
We want users to trust and adopt our AI tool, so Qualtrics Assist’s voice must:
Facilitate trust building,
Influence the user’s willingness to adopt the tool, and
Build stronger emotional connections and prompt action.
PERSONA
They oversee consumer strategy and insights for a major media company, using Qualtrics Assist to analyze customer feedback.
The VoC analyst.
I need clear summaries of my data to reduce cognitive load and help stakeholders understand the main issues.
But the responses are often unclear, unspecific, or lack nuance. I have to ask questions like, “In human speak, what are people saying about this specific category?”
“
After endless Googling, there weren’t enough resources for my exact use case. I kept running into voice guidelines for brands and support bots, and I couldn’t find what I needed.
To at least get started, I applied our tone of voice to the Four Dimensions of Tone of Voice.
RESEARCH
Formal—Casual: Generally conversational, but we don’t push it.
Serious—Funny: Our clients are highly educated professionals who use Qualtrics for work, and our language is tailored this way. There aren’t many opportunities to be humorous here.
Respectful—Irreverent: We treat users with the utmost respect.
Matter-of-fact—Enthusiastic: Context-dependent — if we’re giving kudos, we’ll be enthusiastic. But we’re mostly matter-of-fact.
If we’re already not humorous or irreverent in our product, we shouldn’t experiment with these as it wouldn’t build trust.
In fact, our target audience might distrust us.
DEFINING VOICE, TONE, FORMALITY, AND LENGTH
SKETCHES: FORMALITY, LENGTH, AND TONE
I presented this to Terry Anderson (senior XM scientist), Claudia Martinez (lead designer), and Marc Hannum (head of content/UX).
FEEDBACK
Similarly, we researched how users reacted to informational or supportive tones in other AI features (automated summaries, AI-generated comments). Here’s what we found:
Users strongly prefer the AI to be objective when working with data analysis
Emotionally charged (or “cheery”) tone is considered irritating and inappropriate from an AI
Participants want AI summaries to “get to the point” and “tell me what I need to know”
Concerns with how the AI handles survey respondents that used informal language or slang
Participants want to be met where they are, rather than forcing a one size fits all conversation style
CHANGES
Celebratory: Not an option; it’s the default success tone
Educational: For users wanting to learn more about their insights
Encouraging: Not an option; may be emotionally charged
+ Helpful: For users out of their depth — often first-timers
Informational: For users who just want the facts and next steps
Inspiring: Not an option; may be “cheery” and irritating
Supportive: Not an option; may be emotionally charged
Tone, revisited
Casual: Not an option; may be too informal and lack nuance
Neutral: Default formality, not a setting
Formal: Not an option; no need for it
Formality
Length
Expanded the maximum lengths and added two options.
+ Brief → <500 characters
Short → <750 characters
Medium → <1,250 characters
Long → <1,750 characters
+ Extended → <2,500 characters
RESULTS
Guidelines ——
In-product ——
I designed, prototyped, and wrote the content for this entire flow.
I really applaud her for her impact to [Qualtrics Assist] — which is pushing the designs forward [and] generally punching well above her level. Out of all of the L3s I have worked with, Sarah is by far the strongest designer, systems thinker and problem solver.
Here’s what the lead designer had to say:
“
De Visser, E. J., Monfort, S. S., Goodyear, K., Lu, L., O’Hara, M., Lee, M. R., ... & Krueger, F. (2017). A little anthropomorphism goes a long way: Effects of oxytocin on trust, compliance, and team performance with automated agents. Human factors, 59(1), 116-133.
Dorton, S. L., & Harper, S. B. (2022). A naturalistic investigation of trust, AI, and intelligence work. Journal of Cognitive Engineering and Decision Making, 16(4), 222-236.
Kaplan, A. D., Kessler, T. T., Brill, J. C., & Hancock, P. A. (2023). Trust in artificial intelligence: Meta-analytic findings. Human factors, 65(2), 337-359.
REFERENCES
prev / next